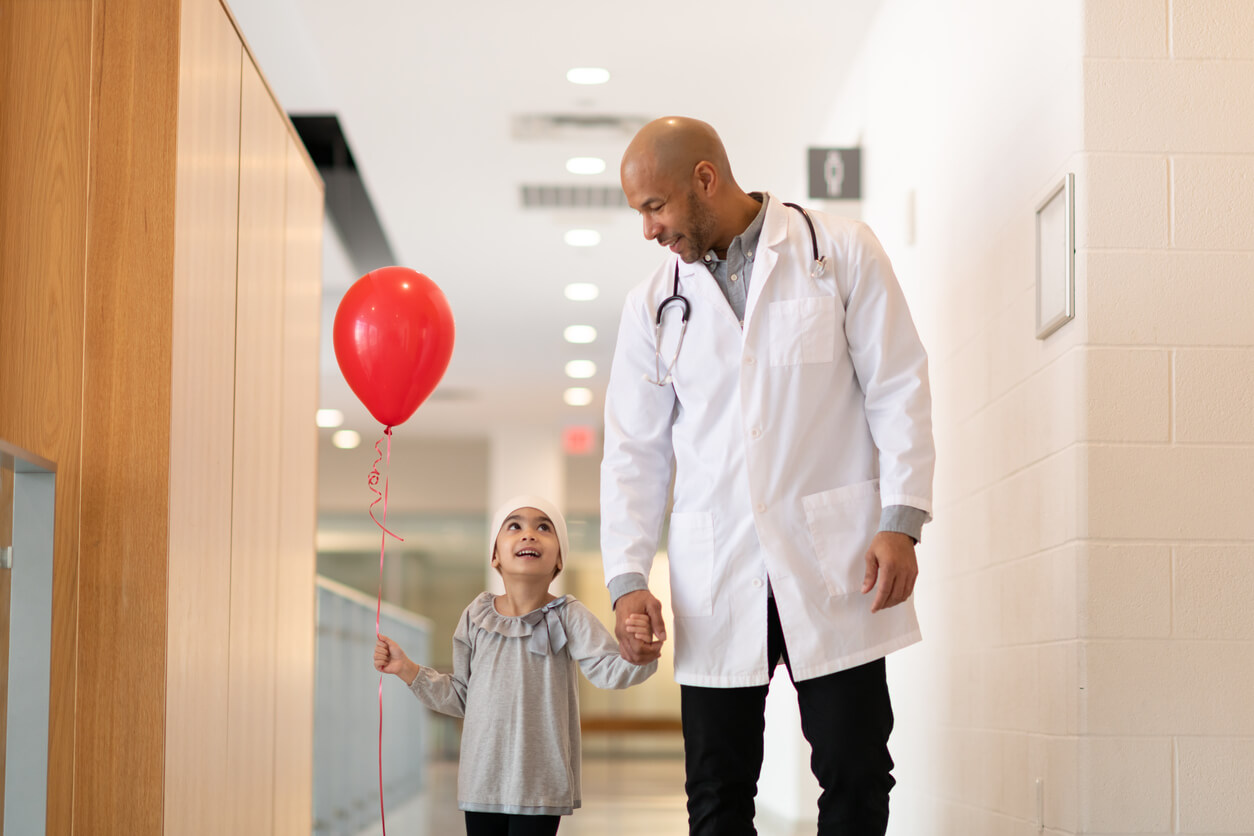
The goal of value-based care initiatives is complementary to the framework of the Triple Aim established by the Institute for Healthcare Improvement (IHI).
CMS Triple Aim Framework
- Better care and experience for individuals
- Better health for populations
- Lower per capita costs
In order for health plans and providers to achieve these goals, organizations need access to relevant, timely and meaningful data. Once they have this data in hand, they need a way to mine it for relevant trends and insights to make more informed decisions about where to invest their capital, time, and resources to drive change.
Today, artificial intelligence (AI) techniques are being used to help healthcare organizations make sense of vast amounts of data and equip them to succeed under value-based arrangements.
AI Applications: Better Care & Experience For Individuals
This component of the Triple Aim has some roots in the Institute of Medicine’s Crossing the Quality Chasm initiative, which emphasized the need for healthcare to be safe, effective, timely, efficient, equitable, and patient-centered.
AI is being utilized to bring insights about the patient into clinical care and decision-making. The insights gathered through the use of AI about the individual includes:
- indications of the patient’s underlying risk factors
- care needs
- social determinants of health
- their experience with the healthcare ecosystem
This is gleaned from structured and unstructured data sources. With this information in hand, the health system or plan can anticipate and close gaps in care or appropriately address social determinant factors unique to each patient.
While the goal of providing better care to individuals is an important one, it’s difficult to measure and quantify. There are a number of tools in the market to capture patient experience, such as Net Promoter Score (NPS) surveys, the Health Outcomes Survey (HOS), the Consumer Assessment of Healthcare Providers and Systems (CAHPS), and other patient-centered survey tools.
Patient surveys and interviews can capture useful feedback on where plans and providers can improve their services, but these formats can be challenging to distill into actionable takeaways. Partially, this is because the most valuable qualitative patient feedback data is captured in free-form text fields that traditional analytic techniques can’t do much with. Partially, it’s difficult to read between the lines of patient responses, identifying bias and defining real issues around individual preferences.
AI techniques can be used to address some of these patient experience data challenges. Machine learning classifiers can be trained to mine free-form survey responses and audio content to look for key themes or dimensions of care—for example, a classifier could aggregate mentions of a topic related to a potential business initiative a healthcare organization may be considering. It could also be used to analyze word choices in free-form responses to infer sentiment or bias to help an organization accurately interpret patient feedback. By getting more targeted insights out of every patient touchpoint and filtering out noise during analysis, AI can help healthcare organizations get a clearer read on what makes their patients happy, where they can improve clinical care and customer service, and what additional support or resources can help their patients stay healthy.
AI Applications: Better Health For Populations
Managing population health is a critical component of value-based care—but it’s no easy task. Understanding broader patient health needs within a target population, defining care gaps that impact patient health, mining social determinants data to tailor care plans, and routing patients to the appropriate care and case management programs can be challenging.
There’s a wealth of clinical and operational data that can be mined for population health initiatives from claims, EHRs or patient charts, labs, biometrics, and third-party socioeconomic data sources. However, to date, most value-based care programs have struggled to fully access and utilize this data to inform decision-making. It is estimated that 80% of available healthcare data is unstructured and underutilized. The volume of available data is both a blessing and a curse: A blessing in that it has the power to reveal important trends and insights about patient health and risk factors; a curse in that it’s way too much content to analyze through traditional means.That’s where AI can help.
AI makes sense of large, ever-changing healthcare datasets by surfacing key pieces of information to inform specific tasks. AI is currently being used to support a variety of population health activities. Classifiers can analyze patient records for evidence of conditions that impact risk stratification. Machine learning algorithms can mine clinical and SDoH data to surface current and prospective health needs to inform disease and care management as well as other population health programs. Other algorithms can be applied to claims and clinical data to analyze provider diagnostic, treatment, and prescribing patterns for potential performance issues that impact patient health. If acted upon, these AI-generated insights can improve clinical care and patient outcomes, positively impacting overall population health.
AI Applications: Lower Cost Per Capita
Value-based care programs aren’t just about improving health—they’re also designed to streamline costs. Many value-based contracts incorporate shared downside risk or capitation payments that incentivize cost savings on the provider side. However, cost efficiency is an ongoing challenge as the price of drugs, durable medical equipment, and treatments continue to rise and population health needs increase.
To contain costs and prevent wasteful utilization, plans have implemented checks and balances such as prior authorizations and formulary requirements. But these processes add administrative burden to provider workflows and can often delay necessary patient care.
There’s a wealth of cost-related data available to value-based care programs: claims data on the billed costs of services, drugs, and equipment; list prices for drugs and DME; and negotiated pricing for plans and providers. AI can be used to make sense of this data and identify areas for cost improvement. For example, AI could be used to search patient EHRs to confirm medical necessity for procedures or drugs that require prior authorizations, analyze claims to find utilization patterns that are potentially wasteful, and identify chase lists of patients with suspected conditions that may need further diagnostic testing or treatment to avoid costly downstream complications.
AI can’t solve every value-based care delivery challenge, but it’s a worthwhile investment for organizations that want to use data-driven insights to improve the health of their populations, contain care costs, and provide a great patient experience under value-based contracts.
Artificial Intelligence For Value Based Care
Apixio provides value-based model healthcare technology solutions for providers looking to implement the CMS Triple Aim Framework.
Our Triple Aim solutions
- AI for better care and experience for individuals
- AI population health management platform solution
- AI to lower per capita costs
Curious about how AI can help your organization succeed at value-based care? Explore Apixio’s AI-powered solutions for risk, prospective, and quality.