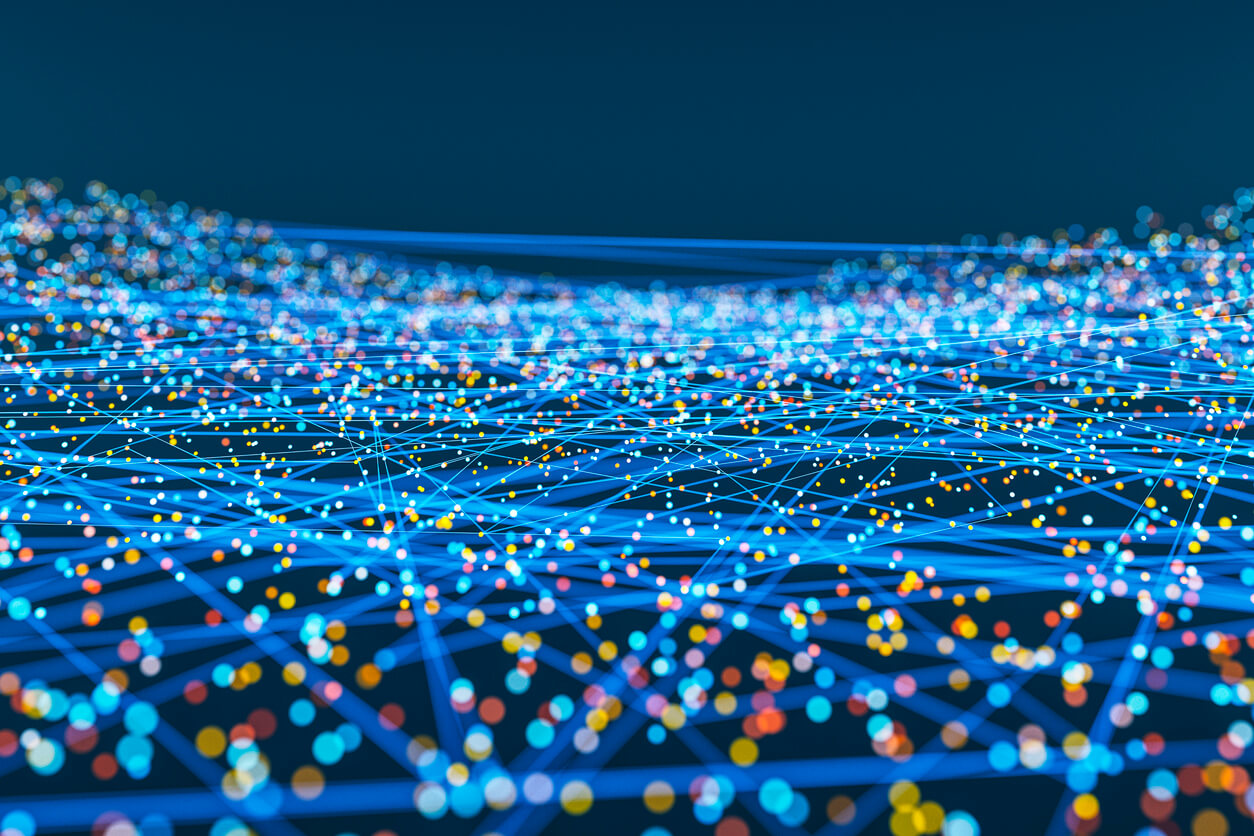
Artificial intelligence (AI) is a hot topic in healthcare today. Every trade show, industry publication, and research journal is talking about the potential applications and benefits of these technologies for payer and provider organizations. But all of this buzz can be difficult to navigate with the myriad different terms, descriptions, and use cases described in various settings.
This primer is designed for healthcare decision makers who need a foundational understanding of AI techniques and applications to keep up with industry news and thoughtfully evaluate potential vendor solutions utilizing these technologies.
Artificial Intelligence (AI)
Definition
Artificial intelligence (AI) is a collection of computational techniques that aim to simulate human behavior and decision-making. AI is the broad category under which specific techniques fall, including machine learning, natural language processing, deep learning, and others. AI is also referred to as cognitive computing.
There are two main types of AI in development today:
- Narrow AI, which is designed to perform specific tasks. All widely-adopted AI applications in the market today are some form of narrow AI.
- General AI, which is designed to perform any task as well as a human. To date, most general AI has been largely research-oriented, and has not been released for general consumption. One notable exception is IBM Watson, which has been applied to everything from personalized advertising to financial governance to drug discovery with varying degrees of success.
What It’s Good For
AI is good at uncovering patterns and insights in large amounts of data, classifying specific pieces of information, and making predictions based on perceived trends—and doing this work more accurately, quickly, and cost effectively than humans. For example, Google search algorithms are really good at analyzing search terms, user demographic information, web content, and feedback from billions of searches performed through its engine to discern patterns and predict which pages will be most relevant to a given query.
Applications in Healthcare
Over the past few years, AI has been applied to a wide range of healthcare areas, including:
- Healthcare operational tasks such as billing, documentation, risk adjustment coding, and quality measurement.
- Clinical activities such as diagnostics, condition suspecting, surgical techniques, and precision medicine.
- Patient engagement programs that involve care management and call center routing.
Natural Language Processing (NLP)
Definition
Natural language processing (NLP) is a set of techniques that help computers process language the way humans do. This includes extracting meaning from written text or speech, and generating readable, grammatically correct original content.
There are two core types of NLP: rules-based NLP, which relies on a manually-programmed dictionary of rules, and machine learning-based NLP, which uses algorithms to develop its own set of rules to best classify information. Rules-based NLP is more common, but also more limited in use cases and insight generation.
What It’s Good For
NLP can be applied to any use case that involves written or spoken language. There are many consumer applications of NLP in the market today, including voice-enabled assistants like Siri and Alexa, semantic search engines like Google and Bing, email spam blockers, and spell check / grammar tools.
Applications in Healthcare
Clinical documentation happens in written and spoken words, which means there are many potential applications of NLP in healthcare. Today, NLP is used to:
- Assist doctors in taking better notes at the point of care via clinical documentation improvement (CDI) solutions.
- Flag duplicative or potentially fraudulent claims submitted for payment.
- Provide customer support for members via chatbots.
Machine Learning (ML)
Definition
Machine learning (ML) is a type of artificial intelligence that focuses on automated knowledge acquisition. The goal of machine learning is to use algorithms to learn, recognize patterns, and solve problems without explicitly programmed instructions.
Machine learning works best when you have a large, clean data set to analyze and a consistent set of training data to “teach” the system how to recognize patterns. For certain types of algorithms, user input can also be helpful to further train algorithms with real-time feedback.
What It’s Good For
Machine learning is ideal for classifying information, finding trends in data, and making predictions about future trends. Some real world examples of machine learning in action include Gmail’s email tab filter (classification), Facebook’s inappropriate content blocker (trends), and Netflix’s content recommendations (prediction).
Applications in Healthcare
Machine learning algorithms are used to optimize a variety of healthcare operational and clinical tasks. This technology is helping health plans and providers:
- Mine EHRs and patient charts for data to inform operational and clinical programs.
- Streamline HCC coding and quality measure abstraction processes.
- Develop accurate chase lists for patients with suspected conditions.
- Accelerate drug discovery & personalized pharmaceutical development.
Neural Networks / Deep Learning
Definition
Neural networks (or deep learning systems, as they’re often called today) are a subset of machine learning algorithms designed to replicate the way humans learn. Neural networks start with a set of training data, then modify their own code as they learn, tuning their algorithms over time. This type of machine learning is unique because it uses a large network of interconnected processing elements (called nodes or neurons) arranged in layers that work together to analyze and interpret data. Layers extract specific pieces of information (called features), and each layer builds on the one before it, assembling complex features out of smaller subcomponents.
What It’s Good For
Neural networks are ideal for complex pattern recognition and trend analysis in very large datasets. They’re best suited for systems that generate a high volume of new data, such as social networks, search engines, media streaming companies, and healthcare organizations.
Applications in Healthcare
Neural networks are currently being applied to an array of healthcare areas. This technology is being used to:
- Automate and improve the accuracy of medical imaging analysis.
- Identify potentially harmful medication interactions.
- Mine personal health history trends to inform precision medicine .
- Select the best candidates for clinical trials.
Machine Vision / Computer Vision
Definition
Machine vision (also called computer vision) is a subset of deep learning techniques that allow computers to identify distinct objects, scenes, and activities in visual content. They look at an image and break it into smaller pieces for classification and interpretation.
What It’s Good For
Machine vision is suited for any application that relies heavily on extracting information from photos and videos. Facial recognition apps, autonomous vehicles, Snapchat and Instagram filters, and car backup cameras all use machine vision to extract insights from visual data.
Applications in Healthcare
Since most machine vision AI applications are close to the point of care, the development and adoption curve isn’t as mature as other types of AI. Technology companies are currently building machine vision solutions to help physicians with:
- Analyzing medical imaging (X-rays, CT scans, MRIs, etc.).
- Diagnosing patient conditions using telemedicine.
- AI-assisted surgical techniques (manual and robotic).
When to Invest in AI Solutions for Your Healthcare Organization
Artificial intelligence can be an extremely powerful technique—but only when applied to the right problems in the right way. Here are a few questions your healthcare organizations should explore before considering an investment in AI solutions.
- What problems are we trying to solve? Artificial intelligence is most valuable when you have a clear understanding of the business problems you’re trying to solve. Generally, the more complex or widespread the problem, the more value you’ll get out of implementing an AI solution.
- What are we specifically looking for in our data? To make the most of current AI technology, your organization needs to have a specific idea of what trends, predictions, or categories of information you want to find. Otherwise, it’ll be difficult to train algorithms to effectively surface the insights you need.
- What kind of data do we have access to? Unless your organization has consistent access to large sets of structured and unstructured data, the value of AI will be limited. As noted above, AI algorithms work best when they have a lot of information to process and analyze.
- Is our data quality consistent and reliable? If you don’t trust that your internal data sources are accurate, timely, and consistent, there’s no point in running AI algorithms on top of them. You’ll only end up with skewed results that don’t accurately reflect what’s really happening in your data.
- What’s the track record for this type of AI application? Have other companies implemented AI techniques to solve the problems you’re trying to solve? If so, what results have they had? If your organization is new to AI, it’s best to start with proven solutions to ensure your investment yields positive business results.
Still have questions about artificial intelligence and whether it’s right for your organization? Contact us for more information.