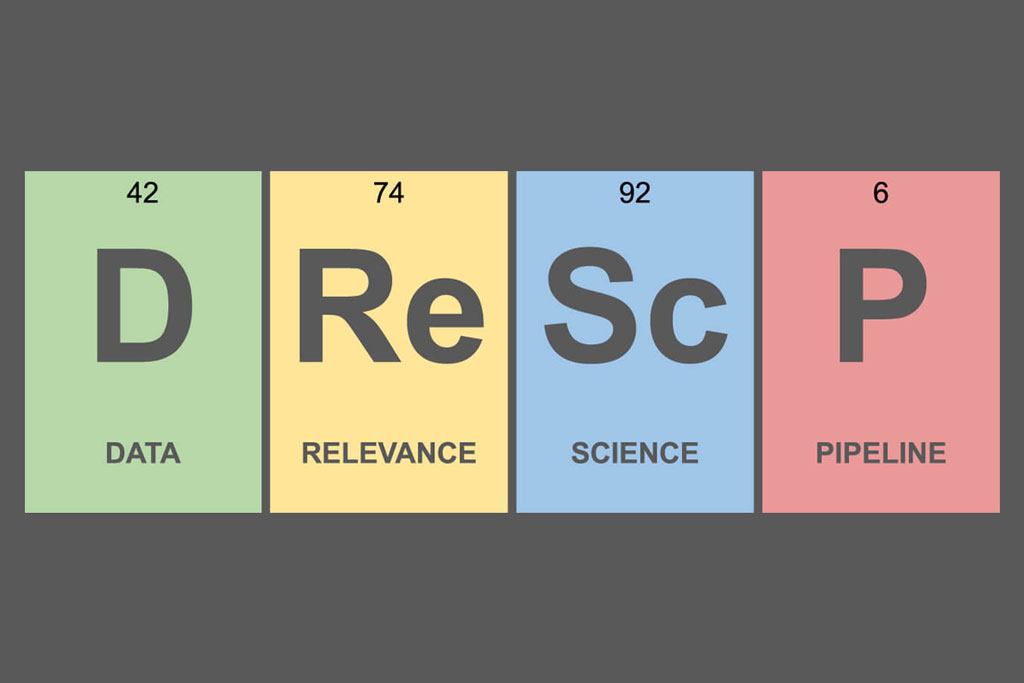
Carbon, hydrogen, oxygen, and nitrogen are four elements essential to life. They form the building blocks that make life possible and account for 96% of all atoms in living things.
Just as there are core elements in nature, there are foundational elements that power the startup world. Successful tech companies share a core set of elements that allow them to create meaningful products and make money selling them. For tech companies who describe themselves as “AI startups,” there are four essential elements for success:
- DATA: The company has reliable access to enough relevant data to train ML models.
- RELEVANCE: The company is capable of creating a bridge between data science and customer value to drive revenue growth.
- SCIENCE: The company has effective algorithms to extract relevant insights from its data.
- PIPELINE: The company has the infrastructure in place to deploy machine learning models at scale.
These elements may seem like natural building blocks, and yet many AI startups today only have one or two in place. A recent report from MMC ventures concluded that among 3,000 startups, only 60% had evidence of AI material to a company’s value proposition, leaving a staggering 40% without.
40% of AI startups do not have evidence of the four essential elements.
Defining the Four Elements of AI Startups
Before startups can implement the four essential elements, they need to have a clear definition for each one and understand how to incorporate them into their strategy.
DATA. The first element consists of getting access to data—quality data in large enough quantities to label and train high-performing ML models. Acquiring this element is particularly challenging where data is difficult to retrieve or highly regulated. Furthermore, as machine learning is becoming more widely-used, startups are facing the challenge of not necessarily having enough data to label. Deep learning techniques also require larger amounts of labeled data than traditional machine learning algorithms.
In order to acquire data, a startup must have the ability to convey a story that contextualizes the data and shows value to their customers—in other words, they must provide a compelling reason for customers to share data with them.
RELEVANCE. This element is about creating a bridge between data science and customer value. It’s a tricky process that involves people from across the business to determine what information or learnings to extract from the data and identify why those learnings matter to the client. Thinking about model (classifier) performance in terms of real world customer outcome helps a company to price their product and demonstrate value to the customer. This element is often an afterthought for many AI startups, but in order to incorporate it effectively, teams must perform upfront research to evaluate what is possible, what the desired insights and predictions might look like, and what problems or needs the insights address.
SCIENCE. This element is related to a company’s ability to leverage research and algorithms to create models that extract insights from data. The model(s) need an algorithm (“how to learn”) and a training dataset (“what to learn from”). Data labeling, model training and evaluation are the main capabilities of the data science element.This is where most companies focus their efforts and where most media outlets spend their time covering innovations.
An AI startup needs to attract top talent for this element, and while the global AI talent pool may be growing, demand still exceeds supply, according to a recent report from Element AI.
PIPELINE. This final essential element for an AI startup is the ability to build, train and deploy machine learning models quickly into production at scale. A machine learning pipeline commonly has the following capabilities:
- Model deployment and verification
- Live data ingest and prediction generation at scale
- Dataset prioritization for dynamically changing business needs
- Active learning
- Continuous outcome verification and auditing
Below is the machine learning model pipeline we have developed here at Apixio.
Dr. Ikhlaq Sidhu, Chief Scientist & Founding Director at the UC Berkeley Sutardja Center for Entrepreneurship & Technology, makes a good point about this emerging element: “When people study, teach, or discuss data, AI, and blockchain applications today, […] it feels like everyone only concentrates on the algorithms and technical capabilities.” However, if companies don’t have scalable pipelines to deploy their models, the overall impact of their data science work will be extremely limited.
AI startups with non-standard or highly regulated data like Uber, AirBnb, and Apixio are building data science pipelines in-house. Others are leveraging emerging machine learning as a service (MLAAS) solutions from Google, Amazon, Microsoft, and IBM. MLAAS solutions can help startups ramp up a pipeline fairly quickly, but they won’t gain a true competitive edge the way they can with a proprietary pipeline solution.
But Wait—There’s One More!
Back when I did due diligence before joining Apixio, I determined that the company had all four essential elements. Check. But there is more to life than survival. Apixio, as it turns out, is an AI startup with a fifth, very rare, element: PURPOSE. Apixio uses AI to gain insights from patient data. The work we do here improves people’s lives and has the potential to transform the broader healthcare industry. That’s something!
__________________________
About the Author
Leo Janze is VP Engineering at Apixio and writes blogs on engineering leadership.